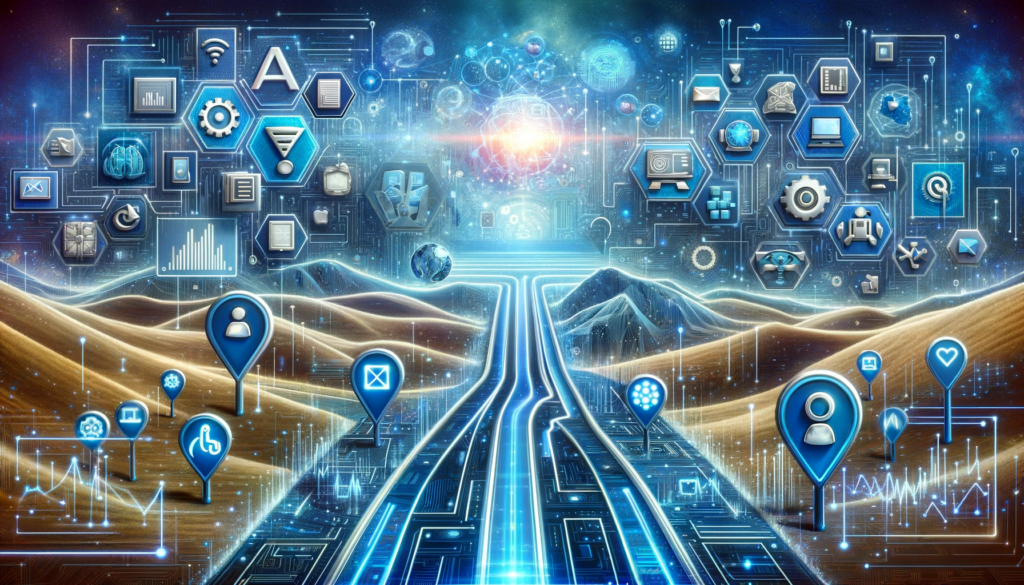
When it comes to selecting an AI productivity tool, it is important to approach the decision with caution and a critical eye. Making the right choice requires careful consideration and a healthy dose of skepticism.
Let’s look at some of the best practices and things you should keep an eye on during your AI tool selection process.
First, let’s talk about data security and privacy. Like a trusty friend, a good AI tool needs to respect your secrets. Some AI tools might store your data or use it to train their algorithms. It’s essential to understand a tool’s data policies before you start working with it. Imagine you’re a doctor, and you’re using an AI tool to handle patient records. You wouldn’t want those records getting into the wrong hands, would you? Always prioritize data security and privacy.
- Data breach: If the AI tool stores your data on insecure servers or lacks robust security measures, it could potentially be breached by hackers. This can lead to sensitive information being stolen and misused.
- Loss of privacy: If the AI tool doesn’t have a good privacy policy or if it uses your data for training its AI, there’s a risk of your private information being exposed. This can include sensitive personal or business data.
- Legal issues: There are numerous laws and regulations about data privacy, such as the General Data Protection Regulation (GDPR) in the European Union. Non-compliance because of using an AI tool that doesn’t follow these laws can result in hefty fines and lawsuits.
- Damage to reputation: A breach of data security or privacy can cause significant harm to an individual’s or business’s reputation. It can lead to a loss of trust among customers or clients, which can impact business relationships and bottom lines.
- Identity theft: In the worst-case scenario, if personal data is leaked, it could lead to identity theft. Criminals can use personal information to commit fraud, causing severe financial and emotional distress to the victims.
Next, it’s crucial to test the quality of the output. Think of it as a taste test for your favorite ice cream. You’d want to make sure the tool can produce high-quality, coherent, and contextually accurate outputs. This is especially crucial when you’re using the tool for important tasks, like an architect using AI to design building structures.
- Inaccurate decisions: If you’re using an AI tool to aid decision-making, and the output is inaccurate, it could lead to incorrect decisions. This can have broad implications depending on the context, ranging from financial losses in business decisions to potentially harmful effects in healthcare settings.
- Wasted resources: If you don’t check the output quality and base actions on poor quality results, it may lead to wasting time, money, or other resources on incorrect or ineffective strategies or solutions.
- Loss of trust: If your stakeholders, whether they’re clients, customers, or internal team members, realize that the information generated by the AI tool is incorrect, it may lead to a loss of trust in the tool and your processes.
- Increased risk: In certain fields, especially those dealing with sensitive data or operations, low-quality output from an AI tool can significantly increase risk. For instance, in cybersecurity, an AI tool that fails to accurately identify threats can lead to breaches and substantial damage.
- Legal consequences: In some instances, especially with regulated industries like healthcare or finance, the use of AI tools is governed by strict standards and regulations. If an AI tool produces low-quality or erroneous outputs that lead to non-compliance or harm, it could result in legal repercussions.
Then, check how customizable the tool is. We all have our unique needs and preferences, and the AI tool should be able to meet those. Relying solely on vendor demos is like judging a book by its cover. Some AI tools can mislead users to think they can do magic when they’re really pulling a rabbit out of a hat. So, always test the tool on cases that closely resemble your specific needs.
A good example is an AI tool being evaluated for the task of analyzing medical images for early detection of certain diseases. Vendor demos might showcase the tool’s abilities using a pre-selected set of images under ideal conditions. These images might be clear, high-resolution, and contain obvious signs of the disease, thus leading the tool to identify them accurately. However, in the real-world setting, the conditions are rarely ideal. The images may vary in quality due to different equipment, patient conditions, and imaging techniques. Also, early-stage disease markers might be subtle and not as apparent as in the demo set.
So, a hospital decides to test the tool on their own set of anonymized images representing real-world use cases. The test set includes both high-quality and lower-quality images and represents a range of disease stages. By doing so, the hospital can better evaluate the tool’s performance. They find that while the AI tool performs well on high-quality images, its performance drops on lower-quality images. However, knowing this upfront, they can implement processes to ensure that the images fed into the AI tool meet a certain quality standard. The tool still proves to be valuable because it can correctly identify early-stage disease markers that were often missed in manual reviews, even on lower-quality images.
Lastly, measure the tool’s adaptability. The best AI tools are like a good pair of jeans – they get better with time. These tools are designed to learn from user interactions and adapt over time. Even if they seem to perform poorly at first, they have the potential to better meet and anticipate your unique needs the more you use them, just like your favorite jeans molding perfectly to your body over time.
Let’s consider an example from the field of customer service. A company introduces an AI-powered chatbot to handle customer queries. In its initial stage, the chatbot is programmed with a set of predefined responses to anticipated customer queries. However, it’s built with an adaptive learning mechanism, which means it can learn and improve over time based on user interactions.
During the first few weeks, the chatbot might struggle with complex queries or nuanced language and slang. It might provide incorrect responses or fail to understand the customer’s problem entirely. However, because of its adaptability, it’s constantly learning from these interactions.
Over time, the chatbot becomes more skilled at understanding a wider range of queries, including complex ones. It starts recognizing and understanding the slang and nuanced language used by the customers. It learns to predict the type of queries that customers might have based on the context of the conversation and prepares responses accordingly.
After a few months, the chatbot is not only able to handle the majority of customer queries accurately and efficiently, but it also starts to anticipate common questions and provides relevant information proactively. This reduces the response time and improves customer satisfaction.
This scenario shows the positive impact of measuring and leveraging an AI tool’s adaptability. Despite the initial hiccups, the chatbot was able to improve and better meet the needs of the customers due to its ability to learn and adapt from user interactions.